Improving The Capabilities Of Cognitive Radar & EW Systems
By Tim Fountain and Leander Humbert, Rohde & Schwarz
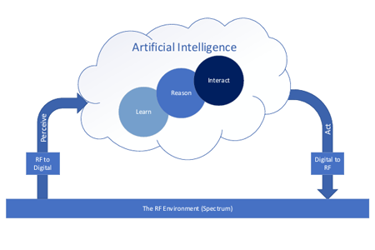
The rapidly evolving landscape of electromagnetic warfare (EW) and radar systems presents significant challenges, particularly with the emergence of mode-agile or Wartime Reserve Mode (WARM) threat emitters. These sophisticated emitters can outmaneuver traditional static threat library implementations, which rely on predefined, repetitive parameters for threat identification and countermeasure deployment. In this paper, we explore the limitations of static threat libraries in the face of such dynamic threats and propose the integration of cognitive Artificial Intelligence (AI) and Machine Learning (ML) systems as a solution. We delve into the architecture of these advanced cognitive systems and demonstrate how wideband RF record, simulation, and playback systems can be utilized to train AI/ML engines.
By evaluating the effectiveness of these countermeasures on real hardware, we aim to illustrate the potential of cognitive RF systems to autonomously perceive, learn from, and adapt to their environments, ensuring robust electronic attack (EA), electronic protect (EP), and electronic support (ES) capabilities. This paper will discuss the technical challenges and solutions associated with implementing these systems, emphasizing the importance of continuous adaptation and real-time response in modern electronic warfare.
Get unlimited access to:
Enter your credentials below to log in. Not yet a member of RF Globalnet? Subscribe today.