NASA's Plan To Make Sense Of Roman's Massive Amount Of Data

By John Oncea, Editor
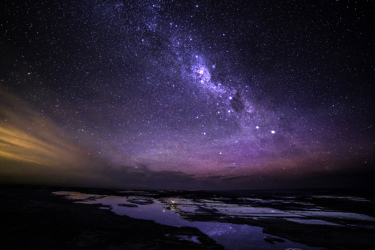
The Nancy Grace Roman Space Telescope will use algorithmic tools to find hidden signals in space, including supernovae and exoplanets.
The Nancy Grace Roman Space Telescope (Roman), scheduled to lift off in the spring of 2027, is named after the “mother of the Hubble Space Telescope.” With a field of view at least 100 times higher than Hubble’s, Roman has the potential to measure light from a billion galaxies in its lifetime.
This capability, along with the ability to block starlight to directly see exoplanets and planet-forming disks, will enable Roman – which we introduced on our sister site, Photonics Online – to meet its objective of settling essential questions in the areas of dark energy, exoplanets, and infrared astrophysics.
Meeting those objectives is going to require Roman to collect unprecedented amounts of light curve data that hold clues to new planets, supernovae, and other astrophysical phenomena. Hidden within this vast sea of data are signals that could lead to groundbreaking discoveries, writes TechPort.
How To Sift Through All That Data
To help make the voluminous amounts of data Roman is expected to produce more accessible to researchers, NASA’s Goddard Space Flight Center created a project to develop a universally applicable, computational, machine-learning-assisted framework to help in identifying known or predicted astrophysical signals in Roman’s light curve data.
Researchers worked on the project from May through September 2024, generating mock data and training an advanced neural network to make it easier to sift through massive datasets without requiring large-scale data analysis.
“The primary obstacle to the exploitation of science from optical light curve survey data is the researcher’s ability to find a light curve containing the astrophysical signal in which they are interested from tens to hundreds of millions of light curves,” TechPort writes. “This obstacle will only grow as the size of light curve data sets is augmented by improved observatories such as Roman.”
NASA researchers set out to form a pipeline using artificial intelligence (AI) and machine learning (ML) that would allow a user to input a theoretical signal, which is then injected on a pre-collected set of template light curves designed to account for noise and systematics. This process creates mock data to be used to train a pre-constructed neural network, needing only minimal training due to transfer learning from pre-trained weights, to classify the astrophysical signal.
“The project was successful in achieving the stated objectives,” writes TechPort. “We created several million synthetic Roman light curves with many different types of modeled stellar variability. We then developed and trained an autoencoder neural network to identify latent features in the light curves and, replacing the decoder with a classification-type neural network, demonstrated the effectiveness of using the encoder as a foundation for fast training of an adaptable classifier.”
The structure and pre-trained weights of the neural network can now be used for implementation in a community-serving code to discover user-defined signals from among Roman light curves upon their release.
Previewing Roman’s View Of The Universe
While Goddard researchers focused on using AI and ML to analyze Roman’s expected light curve data efficiently, Michael Troxel, an associate professor of physics at Duke University in Durham, NC, was leading a simulation campaign of his own.
“We used a supercomputer to create a synthetic universe and simulated billions of years of evolution, tracing every photon’s path from each cosmic object to Roman’s detectors,” said Troxel, according to NASA. “This is the largest, deepest, most realistic synthetic survey of a mock universe available today.”
OpenUniverse, a NASA-led project, used the now-retired Theta supercomputer at the DOE’s Argonne National Laboratory to simulate the vast datasets expected from Roman. The supercomputer processed in nine days what would take over 6,000 years on a regular computer, producing a 400-terabyte dataset that previews observations from Roman, the Vera C. Rubin Observatory, and ESA’s Euclid mission.
Using state-of-the-art physics modeling and real galaxy catalogs, the simulations cover 70 square degrees of the sky – more than 300 full moons – spanning over 12 billion years. Scientists aim to use these data to explore cosmic mysteries, including dark matter and dark energy, by studying how they shape the universe. The dataset contains 100 million synthetic galaxies, allowing researchers to analyze galaxy formation and evolution.
Repeated mock observations enabled the creation of cosmic “movies” showing supernovae as they explode across the simulated sky. These allow astronomers to map the universe’s expansion and develop an alert system to track real-time cosmic events when Roman begins operations. Given the immense data volume, teams are also creating ML algorithms to identify significant astrophysical phenomena efficiently.
“Most of the difficulty is in figuring out whether what you saw was a special type of supernova useful for mapping the universe’s expansion or something almost identical but irrelevant,” said Alina Kiessling, a research scientist at NASA’s JPL and principal investigator of OpenUniverse.
Roman will revolutionize space-based infrared and optical astronomy, surpassing previous telescopes in data volume. “In less than a year, Roman will complete surveys that would take Hubble or James Webb a thousand years,” said.
Scientists will use these synthetic datasets to plan observations and refine data analysis before real Roman observations begin in 2027. Comparing real and simulated data also will test the accuracy of current cosmological models, potentially revealing new physics. “If we see something that doesn’t quite agree with the standard model of cosmology, we must confirm it's truly new physics and not a data misinterpretation,” said Katrin Heitmann, deputy director of Argonne’s High Energy Physics division.
OpenUniverse is a collaborative effort involving NASA’s JPL, DOE’s Argonne, IPAC, SLAC, and several U.S. universities, working alongside the Rubin LSST DESC and Roman Science Operations teams. These efforts will ensure scientists are prepared to harness the transformative potential of Roman’s unprecedented dataset.