Is Artificial Intelligence Coming For Your Job?

By John Oncea, Editor
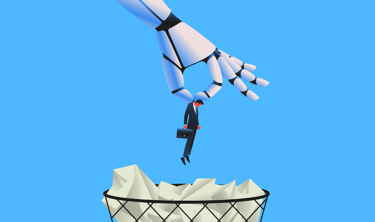
AI can enhance, not replace, an RF engineer by automating tasks but the engineers' creativity and judgment remain vital for complex challenges.
Since OpenAI launched ChatGPT in late 2022, nearly every industry has been transformed in some way, shape, or form. It staked its claim as the fastest-growing consumer application in history, estimated to have reached 100 million active users in just a couple of months while simultaneously spurring an AI arms race amongst dozens of companies.
ChatGPT – as well as other artificial intelligence (AI) driven technologies such as chatbots, machine learning, and the Internet of Things (IoT) – is changing the engineering landscape and promises to provide more disruption in the coming years.
But is AI coming for your job?
Like all nascent technologies, the future of AI and its impact on engineers is hazy at best. But as we dip our toes in the AI era, let’s do our best to try and determine if AI is an engineer’s friend or foe.
A ChatGPT Experiment
As a thought experiment, I entered, “Is artificial intelligence going to replace RF engineers” into ChatGPT-3.5, and here’s the answer:
Artificial intelligence (AI) has the potential to automate many tasks traditionally performed by engineers, including RF (Radio Frequency) engineers. However, it's unlikely that AI will completely replace RF engineers in the foreseeable future. Here are a few reasons why:
- Complexity of RF Engineering: RF engineering involves a deep understanding of electromagnetics, signal processing, and circuit design, among other disciplines. While AI can assist in optimizing certain aspects of RF design and analysis, it may not have the creativity and problem-solving ability of human engineers when it comes to dealing with complex or novel challenges.
- Human Judgment and Creativity: RF engineering often requires human judgment and creativity, particularly in situations where there are trade-offs between different design parameters or when unexpected issues arise. AI may struggle to replicate the intuitive decision-making process of experienced engineers.
- Integration with Other Disciplines: RF engineering is often integrated with other fields such as telecommunications, electronics, and networking. Human engineers are better equipped to understand and navigate these interdisciplinary connections, whereas AI may be limited by its narrow focus on specific tasks.
- Regulation and Safety: RF systems often operate within regulated environments with strict safety requirements. Human engineers are responsible for ensuring that RF systems comply with regulations and safety standards, which may involve complex legal and ethical considerations that AI is not yet capable of handling autonomously.
First, I don’t think AI is quite ready to replace real, live, human writers so, yay for me.
Second – concerning the response – ChatGPT seems to have provided a pretty decent answer. Let’s look closer look and see if it holds water.
Complexity Of RF Engineering
AI does indeed face challenges in dealing with the complexities of RF engineering, including signal characteristics. For instance, RF signals exhibit unique characteristics due to factors like multipath propagation in urban environments and diffraction caused by high water vapor content in the atmosphere. AI algorithms have yet to adapt to these dynamic ranges, interference, and low signal-to-noise ratios.
The development of covert technologies like passive radar and low probability of intercept waveforms pose challenges, too. AI models need to be more resilient to manage a wide range of signal variations and unexpected scenarios.
Finally, the deployment of RF smart sensor systems in medical diagnostics, autonomous vehicles, defense, and agriculture, the increased use of IoT devices, and complex communications like 5G and WiFi6 are creating a more complex and congested electromagnetic environment that AI struggles with.
To be fully capable of managing these engineering complexities, AI needs to grapple with signal complexities, adapt to covert capabilities, and foster interdisciplinary collaboration.
Human Judgement And Creativity
AI once again comes up short when trying to quantify the nuanced aspects of RF engineering that require human judgment and creativity. For instance:
- Contextual Understanding and Adaptability: Human judgment in RF engineering involves understanding the broader context, considering trade-offs, and adapting to specific scenarios. AI lacks the innate ability to grasp context beyond its training data. It operates based on patterns and statistical correlations, which may not capture the full complexity of real-world situations.
- Creativity and Novel Solutions: RF engineers often devise creative solutions to address unique challenges. AI algorithms excel at optimization and pattern recognition but struggle with out-of-the-box thinking. They rely on existing data and predefined rules, limiting their ability to invent novel approaches.
- Uncertainty and Ambiguity: RF environments are inherently uncertain and dynamic. Human engineers navigate ambiguity, make informed decisions under incomplete information, and adapt when faced with unexpected conditions. AI models struggle with uncertainty, especially when encountering scenarios not encountered during training.
- Ethical and Social Considerations: Human judgment incorporates ethical, legal, and social factors. AI lacks inherent ethical reasoning. Decisions made by AI systems may inadvertently perpetuate biases or have unintended consequences. Balancing technical efficiency with ethical implications requires human judgment.
- Intuition and Heuristic Knowledge: Experienced RF engineers rely on intuition and heuristics developed over years of practice. AI lacks this tacit knowledge. It cannot intuitively weigh pros and cons, prioritize certain parameters, or make intuitive leaps based on subtle cues.
- Complex Trade-offs: RF design involves intricate trade-offs: power consumption vs. range, latency vs. throughput, spectral efficiency vs. interference resilience. AI struggles to optimize across conflicting objectives without clear guidance on priorities.
- Interdisciplinary Collaboration: Creativity often emerges from interdisciplinary collaboration. RF engineering integrates physics, mathematics, signal processing, and domain-specific knowledge. AI models may lack expertise in all these areas, necessitating collaboration with human experts.
While AI can enhance certain aspects of RF engineering, it remains complementary to human judgment and creativity. The constructive interaction between AI and human expertise holds immense potential for advancing wireless technologies.
Integration With Other Disciplines
AI faces challenges when merged with other RF engineering disciplines including material platforms and integration methods. Photonic integration has propelled microwave photonic technologies to new heights creating AI challenges such as interfacing hybrid material platforms, enhancing light-matter interactions, and integrating components like modulators and detectors into a single chip. While AI brings immense potential to RF engineering, successful integration requires addressing material challenges, understanding nonlinearities, and fostering interdisciplinary collaboration.
Regulation And Safety
Among the challenges AI faces when integrating it with RF engineering, understanding regulations and safety might be the most important. Here are a few reasons why:
- Safety Engineering for AI: Ensuring safe AI behavior is crucial. Unlike cybersecurity, which primarily focuses on defending against external attacks, AI safety involves internal behavior. Standards and guidelines for AI development are essential but consensus on practical guidelines is still evolving. Industries like aerospace and medical manufacturing offer risk-management models that could serve as a foundation for AI safety standards. Incorporating safety into design thinking and the entire engineering process is necessary. Waiting until after product success to address safety can lead to irreversible consequences.
- Understanding User Needs and Values: Engineers must go beyond surface-level use cases and understand users’ values and preferences. The value-alignment problem highlights the need to dig deeper. For instance, understanding why a student attends school involves more than just gaining knowledge — it includes factors like credentials, peer approval, and earning potential.
- Interdisciplinary Collaboration: Integrating AI with RF engineering requires collaboration across disciplines. Ethical considerations, legal implications, and social impact must be addressed. Engineers need to balance technical efficiency with ethical responsibility.
Again, while AI offers immense potential in RF engineering, addressing safety, regulations, and interdisciplinary collaboration is essential for responsible and effective integration.
Humans By A Nose
There seems to be a consensus that AI is not capable of replacing engineers at this time. It is, instead, expected to automate some of the more mundane tasks, freeing up engineers to focus on more complex and/or creative work. This combination of AI and living, breathing engineers is expected to result in increased productivity and efficiency.
So, while AI will certainly change the nature of engineering jobs, it’s not expected to make those jobs obsolete. It could be quite the opposite where AI makes engineers more valuable due to their ability to judge, regulate, and ensure the safety of the data and concepts AI is producing.
For example, engineering involves complex tasks that require a deep understanding of electromagnetics, signal processing, circuit design, and system integration, among other disciplines. AI can certainly assist engineers by speeding up certain design processes, optimizing parameters, and even aiding in troubleshooting but human expertise is still crucial for tasks such as problem-solving, innovation, decision-making, and understanding the broader context of engineering projects.
Additionally, RF systems often operate in environments where real-world constraints, such as interference and environmental factors, need to be considered. Engineers are needed to interpret and apply AI-generated insights within these contexts effectively.
Long story short: AI is a tool that can assist engineers, not replace them.