Beyond Traditional RF Simulation: 7 Trends Driving Modern Design Software

By John Oncea, Editor
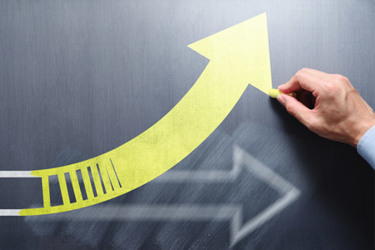
Modern RF design software integrates AI automation, cloud HPC, and multi-technology workflows to address 5G/6G demands and heterogeneous integration complexity.
The rapid evolution of wireless communication technologies, from 5G networks advancing toward 6G and the proliferation of millimeter-wave applications, has created unprecedented demands on RF and microwave design software.
As system complexity increases and integration densities reach new heights, software vendors are responding with transformative innovations that fundamentally change how engineers approach circuit design, simulation, and optimization. These emerging trends represent not just incremental improvements but paradigm shifts that promise to accelerate design cycles, improve accuracy, and enable previously impossible levels of integration.
Multi-Technology Heterogeneous Integration Workflows
The most significant transformation in RF design software centers on seamless multi-technology integration capabilities that address the growing complexity of modern wireless systems. Advanced design environments now provide comprehensive workflows for integrating disparate technologies, including RFICs, MMICs, packaging substrates, printed circuit boards, and antenna structures into cohesive multi-technology modules. This integration challenge has become particularly acute as engineers seek to create compact, high-performance systems that combine multiple semiconductor processes and packaging technologies within single assemblies.
Modern software platforms have evolved beyond traditional single-technology design approaches to embrace full 3D heterogeneous integration workflows. These platforms enable engineers to map different chip technologies onto three-dimensional module assemblies while simultaneously managing connectivity requirements, component orientation constraints, and complex interconnect parasitics. The coordination required for such multi-technology assemblies demands sophisticated design environments that maintain full electromagnetic and circuit simulation fidelity across all integrated components.
The heterogeneous integration approach has become essential for next-generation RF systems where traditional printed circuit board assemblies cannot meet size, weight, and power requirements. Recent industry developments demonstrate the critical importance of this trend, with conferences focusing specifically on integrated innovations that merge software, circuits, and antennas for next-generation interconnected systems, according to the Journal of Industrial Engineering and Applied Science. These multi-technology workflows enable engineers to experiment with different component stacks and orientations while preserving complete model accuracy for electromagnetic and circuit co-simulation.
Python-Enabled Automation and AI Integration
Integrating Python application programming interfaces into RF design software represents a fundamental shift toward automated workflows and artificial intelligence integration. This development enables engineers to programmatically control simulation and optimization processes, creating automated workflows that significantly reduce manual effort while increasing design iteration speed. Python integration extends beyond simple scripting to enable sophisticated interaction with external AI and machine learning frameworks.
Advanced simulation platforms now provide comprehensive Python APIs that make RF design software AI-ready, enabling integration with machine learning models, including artificial neural networks and large language models, Keysight Technologies. This capability allows engineers to leverage external AI algorithms and frameworks, including emerging copilot technologies and AI agents, to achieve unprecedented productivity levels. The Python automation infrastructure enables seamless integration with external synthesis, optimization, and machine learning tools that can accelerate complex design tasks.
The AI-ready architecture facilitated by Python integration represents more than convenience; it provides access to machine learning-driven design optimization, automated parameter sweeping, and intelligent design space exploration. Engineers can now implement custom optimization algorithms, integrate with cloud-based AI services, and develop automated design validation workflows that operate across multiple design domains simultaneously.
Advanced 3D Electromagnetic Simulation Technologies
The evolution of 3D electromagnetic simulation capabilities addresses one of the most computationally demanding aspects of modern RF design. As integration densities increase and operating frequencies extend into millimeter-wave ranges, accurate electromagnetic modeling becomes critical for predicting system performance and avoiding costly hardware iterations. Software vendors have responded with significantly enhanced simulation technologies that provide faster execution times while maintaining high accuracy across complex multi-technology assemblies.
Contemporary EM simulation platforms incorporate multiple algorithmic approaches operating across frequency, time, envelope, linear, nonlinear, stability, electrothermal, system, and measurement domains, writes Purdue University. This multi-domain capability ensures thorough characterization that satisfies diverse specification requirements while providing engineers with comprehensive insight into electromagnetic behavior across all relevant operating conditions. The advanced simulation technologies enable accurate modeling of high-port-count systems with substantially reduced simulation run times compared to previous generation tools.
The enhanced 3D EM capabilities prove particularly valuable for heterogeneous integration applications where multiple technologies with different electromagnetic properties must operate nearby. These simulation advances enable engineers to predict and optimize electromagnetic interactions between RFICs, packaging structures, interconnects, and antenna elements within complex 3D assemblies.
Cloud-Based High-Performance Computing Integration
The adoption of cloud-based high-performance computing represents a change in thinking in how RF engineers approach computationally intensive design tasks. Cloud HPC integration enables engineers to access virtually unlimited computational resources for electrothermal circuit simulations, complex optimization tasks, and large-scale electromagnetic analysis without investing in expensive local hardware infrastructure. This capability proves particularly valuable for the thermal analysis of integrated circuits, where comprehensive modeling requires substantial computational resources.
Cloud-based computing platforms provide scalable access to specialized processors optimized for electromagnetic simulation, circuit analysis, and optimization algorithms, MDPI writes. Cloud integration enables engineering teams to collaborate more effectively while accessing consistent, high-performance computing resources regardless of their physical location. This approach also facilitates more thorough design verification through Monte Carlo analysis, yield optimization, and extensive parameter sweeping that would be impractical with limited local computing resources.
The cloud HPC integration extends beyond simple computational acceleration to enable new approaches to design verification and optimization. Engineers can now perform comprehensive thermal analysis, extensive yield studies, and large-scale optimization tasks that provide deeper insight into design robustness and manufacturing variability.
AI-Driven Circuit Modeling And Optimization
Artificial intelligence and machine learning technologies are revolutionizing circuit modeling approaches, enabling more accurate and efficient model development from measured or simulated data. AI-driven modeling techniques can generate both linear and nonlinear circuit models that capture complex device behavior while providing computational efficiency advantages over traditional modeling approaches. These techniques prove particularly valuable for characterizing new semiconductor technologies and complex multi-physics interactions.
Machine learning algorithms can analyze large datasets from circuit measurements or detailed simulations to extract compact models that preserve essential device characteristics while enabling faster circuit simulation. The AI-driven approach proves especially beneficial for modeling complex nonlinear effects, temperature dependencies, and process variations that traditional analytical models struggle to capture accurately.
Advanced optimization platforms now incorporate AI-enhanced algorithms specifically designed for challenging designs with large-scale variable counts, such as RFICs and 3D heterogeneous integration modules. These optimization approaches can navigate complex design spaces more efficiently than traditional optimization methods, enabling engineers to achieve better performance while reducing design iteration time.
Enhanced Antenna Integration And Placement Tools
The development of specialized antenna integration tools addresses the critical challenge of optimizing antenna placement and performance within complex system architectures. Modern antenna design tools provide comprehensive PCB design capabilities that help engineers choose appropriate antennas, optimize placement locations, and maximize RF performance while minimizing interference between multiple antenna elements. These tools incorporate sophisticated algorithms that enforce adequate spacing between antennas while suggesting optimal placement locations based on electromagnetic modeling.
Advanced antenna integration platforms provide real-time feedback on component placement decisions, enabling engineers to visualize the electromagnetic implications of different antenna locations and orientations, according to Taoglas. The tools facilitate rapid iteration through different antenna configurations while maintaining awareness of mechanical integration constraints and overall system size requirements. This capability proves essential for modern wireless devices that must accommodate multiple communication protocols within compact form factors.
The enhanced antenna integration tools extend beyond simple placement optimization to provide comprehensive performance analysis, including radiation patterns, efficiency calculations, and interference assessment. These capabilities enable engineers to make informed decisions about antenna selection and placement that optimize overall system performance.
Cross-Platform Interoperability And Workflow Integration
The final critical trend involves enhanced interoperability between different EDA platforms and design tools, enabling seamless workflow integration across the complete design process. Modern RF design software provides native compatibility with industry-standard platforms, including Cadence Virtuoso, Synopsys Custom Compiler, and other specialized design environments. This interoperability ensures that engineers can leverage the best capabilities of different tools while maintaining consistent data integrity throughout the design flow.
Workflow-based user interfaces organize simulation and optimization control as coherent workflows rather than disconnected tasks, closely capturing the designer's thought process in producing optimized designs. These workflow-based approaches operate identically across multiple EDA platforms, enabling engineers to apply consistent methodologies regardless of their preferred design environment.
The enhanced interoperability extends to specialized tools for antenna design, thermal analysis, and mechanical integration, creating comprehensive design ecosystems that support the complete product development process. This integration capability proves essential for complex heterogeneous systems that require coordination between RF circuit design, antenna optimization, thermal management, and mechanical packaging.
These seven software trends collectively represent a fundamental transformation in RF and microwave design methodology, moving from traditional single-technology approaches toward comprehensive multi-technology integration platforms enhanced by artificial intelligence and cloud computing capabilities.
The integration of Python automation, advanced electromagnetic simulation, and AI-driven modeling creates unprecedented opportunities for design optimization and verification. As wireless communication systems continue evolving toward higher frequencies, greater integration density, and more complex functionality, these software innovations provide the essential tools needed to meet next-generation design challenges while maintaining the rapid development cycles demanded by competitive markets.